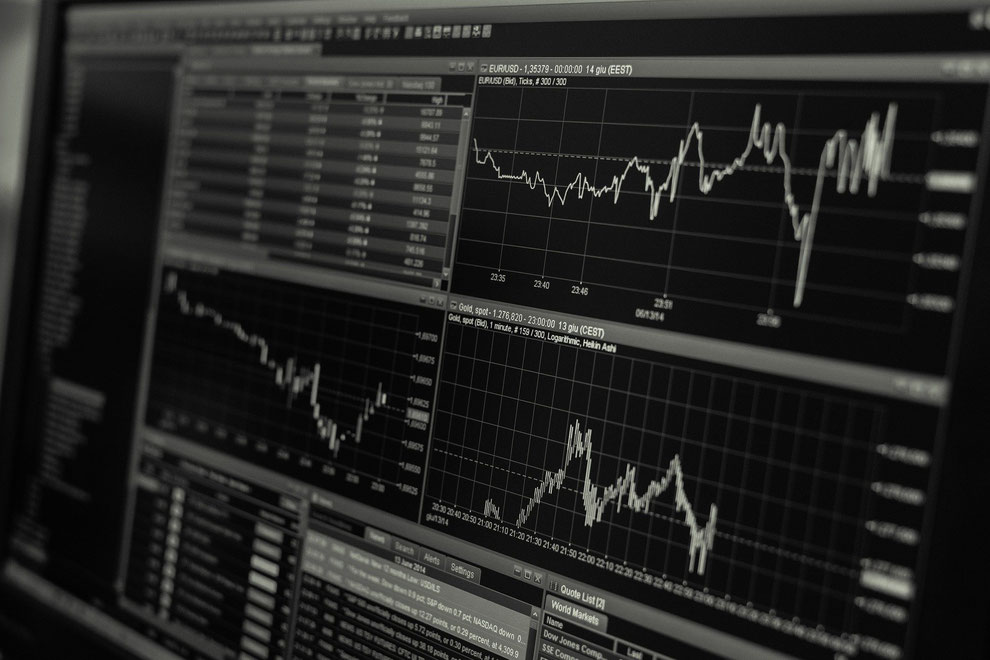
Lorenzo Cafaro from Pixabay
Predictive analytics promise true miracles in many areas, such as industry or medicine. Hopes are therefore high for other areas as well. To what extent can predictive analytics be used for predictions on the capital markets?
The Global Investor magazine interviewed Dr. Christian Brandlhuber, CTO and co-founder of the startup 21strategies, to gain an informed assessment regarding realistic applications of predictive analytics in capital markets. As an experienced specialist, Dr. Brandlhuber has been working in the field of machine learning for over 20 years and is a recognized expert in multi-source/multi-sensor data fusion and "big data" analytics applied to trading tasks. As CTO and co-founder of the startup 21strategies, he has established an artificial intelligence (AI)-based algorithmic decision support engine for CFOs and treasurers that provides situational and strategic predictions under uncertainty for better hedging, for banks for supporting their FX sales staff, for electronic liquidity providers to improve their market making operations, and for institutional investors for more profitable tactical investing or short-term management of liquidity.
According to Dr. Brandlhuber, there is great potential for the use of predictive analytics in capital markets. The prerequisite for success, however, is a meaningful data fusion based on data-driven analyses combined with macroeconomic concepts.
High potential for the use of predictive analytics on capital markets
What are the opportunities and challenges for the successful use of predictive analytics on capital markets? According to Dr. Brandlhuber, predictive analytics have a high potential on the capital market if they are used sensibly. However, the successes in the industrial sector, for example, are not so easy to transfer to the capital market. One reason is that the factors influencing the capital market are more complex. The data is not available in the same quality and density as in other areas. In the industrial environment, thus, the disturbing factors due to the environment are relatively low. The available data is therefore of high quality. In addition, data must also be available in sufficient volume for successful use. In some areas, AI has also been so successful because data was relatively easy to generate. That is, representative data sets with an enormous volume or systems that generate any number of training data via self-play, as in the game of Go. According to Dr. Brandlhuber, the decisive factors are data quality and data density in order to achieve meaningful results.
The complexity of the capital market – a combination of different data types
However, there are fundamental differences to the capital market according to Dr. Brandlhuber. It is true that there is a high number of transactions and thus also of data in quality and quantity. This would be the ideal prerequisite for training large systems. However, there is also relevant information, for example from central banks, which must also be taken into account in intelligent systems. This would often only be available at intervals of several weeks. This is where the real challenge lies: a sensible combination of semantic concepts, market-describing theories, and data-driven approaches. Predictive analytics on capital markets must therefore follow hybrid approaches. Only in this way can the generated data be fused and interpreted in a meaningful way. Another major topic of predictive analytics in the capital market environment is the dynamics to which price formation processes are exposed. A purely statistical approach has only a limited validity in time due to the strong impact on the capital market. This alone is why models that take macroeconomic influences into account are so crucial.
Asset Management: data fusion – the key to success
According to the CTO of 21strategies, there are three major developments in the field of asset management. These mainly include data acquisition, its interpretation and, in a final step, a summary in probabilistic models. The first development direction are applications that are primarily data-driven and can therefore analyze transaction patterns and microstructures in the market. Here, data would be available in the required data density. Another development direction are applications that are primarily aimed at information retrieval and automation of data preparation. These include, above all, analyses of image and text-based sources, especially news. This approach is summarized under the term Named Entity Recognition. The third developments are mainly probabilistic models, as these correlate and interpret the results of the first two approaches. These would exist primarily as strategic risk models.
AI must be based on adapted theoretical concepts
Predictive analytics on capital markets can only be used successfully if models are developed that combine data-driven analysis with macroeconomic models in the sense of a data fusion. These models can only then enable a more systematic and complete view of market risks and opportunities.
According to the CTO of 21strategies, there are hardly any limitations to the use of predictive analytics on capital markets. The prerequisite, of course, is that AI is also used on the basis of theoretical concepts that are tailored to the field of application. Then the advantages of using data in real time could come into play. Purely quantitative systems can thus become more powerful.
Interactions of different AI systems are not taken into account
From an ethical perspective, the CTO of 21strategies advises considering effects and long-term consequences as early as the development of technologies. Risk analyses and the evaluation of possible consequences should go hand in hand with development. Dr. Brandlhuber also draws attention to an aspect that has so far been given too little consideration. Interactions that can occur between interacting AI systems are not given sufficient consideration. This is because systems could also build up on each other if they do not have suitable monitoring. As soon as reinforcement learning systems are used on a broader scale, this aspect will become more relevant.
The full interview with Dr. Brandlhuber on his assessment of the success of predictive analytics in capital markets was published in German in the magazine "Global Investor" on November 12, 2018 under the title: „Predictive Analytics & Kapitalmärkte: Wo stehen wir?“.